Data contributed to AmeriFlux are complex and diverse. Ecosystem-level field sites acquire continuous measurements from many sensors at high temporal resolution, which result in large quantities of data. Data can be collected and processed at different time resolutions and units, and undergo various processes, such as filtering criteria and gap-filling. Data can also be derived through empirical modeling. The team supporting the network, the AmeriFlux Management Project (AMP), works with the field site principal investigators (site PIs) and managers to standardize, quality-check, and process data into forms that the scientific community can use to examine crucial linkages between ecosystem processes and climate responses.
These datasets are free, with the data management costs covered by the U.S. Department of Energy and the Lawrence Berkeley National Laboratory. Site operations are supported by individual agencies and site PIs. Attribution requirements are described in Data Policy.
AmeriFlux Data Products
BADM | AmeriFlux BASE | AmeriFlux FLUXNET | |
---|---|---|---|
What is it? | Biological, Ancillary, Disturbance, and Metadata | The Flux and meteorological time series data | |
Includes gap-filled and partitioned fluxes (GPP, RECO) | No |
Maybe. Included if the site provides these variables. |
Yes |
Temporal Aggregation | Variable | Half-hourly or Hourly | Half-hourly or Hourly, Daily, Weekly, Annual |
Representativeness | Site-level aggregation | All levels of aggregation | Site-level aggregation |
Format | BADM Standards | The FP Standard* |
* Flux-Processing (FP) Standard: standardized variables and format supported by AmeriFlux, fluxnet.org, and other flux networks.
Data Use in AmeriFlux
To download or upload data, you must create an AmeriFlux account. (The same accounts works for FLUXNET or ISCN.)
- Data Policy
- Download Data
- Data Variable Definitions
- Data Variables in AmeriFlux BASE: Data format conventions used within the network
- Data Variables in AmeriFlux FLUXNET (leaving AmeriFlux site)
- BADM—Biological, Ancillary, Disturbance and Metadata
- Data Processing Pipelines: How the AmeriFlux Data Team performs the QA/QC and value-added processing
- How to Upload Data
Data Overview
Data collected at individual sites are sent to an archive operated by AMP on behalf of the network. The AmeriFlux Data Team:
- Performs uniform data format and quality checks
- Aggregates original measurements from individual sensors to ecologically or micro-meteorologically meaningful quantities
- Processes original measurements to provide gap-filled, partitioned, and uncertainty-estimated data products
- Generates and releases high quality and standardized datasets
The standardized datasets facilitate synthesis of earth science information across measurement types, methods of collection, and ecosystems. Scientists use these datasets to assess terrestrial ecosystem responses and feedbacks to the environment, including changes in climate, land use, and extreme events such as droughts, storms or wildfire.
Learn more about the eddy covariance method.
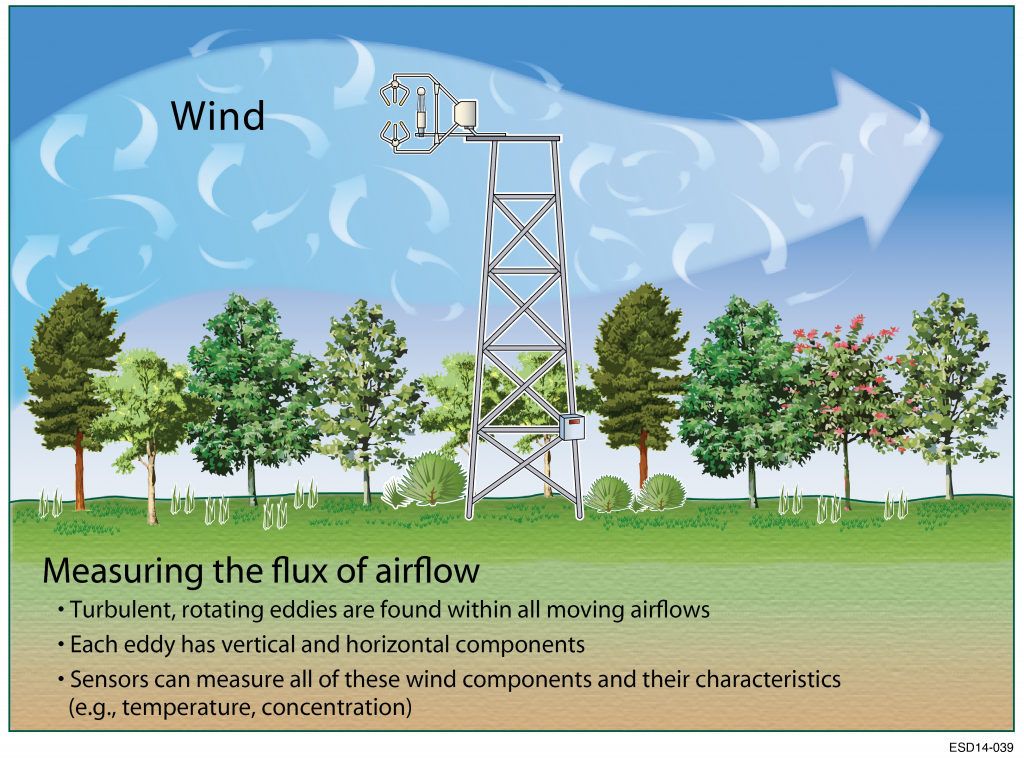